This newsletter uses some recent research to remind us that stress is never good. The first one reviewed is from NY on conventional operations. The remaining 2 are from Europe using robotic milking systems, with activity collars. The studies are linked because they show the potential impact of changes on performance. Control what you can control, because things can, and will, go wrong.
Study #1
Cherney, DJR, et al. 2021. Day-to-day variation in forage and mixed diets in commercial dairy farms in New York. Applied Anim Sci. 37:11-20.
The study was designed to assess variation in daily and weekly silage/haylage dry matter (DM) and nutrients. This study sampled forages and TMR for 4-6 months on 7 commercial dairies in central and western NY. Herds ranged from 300 to 3,000 cows. The main take home point is that more frequent DM determination is critical for reducing the variation observed between the formulated ration and the ration consumed. The authors used a range of 7 points as being important enough to consider adjusting ingredient moisture.
Put another way, how often during any given time frame does moisture move more than 3.5 points, up or down? During this study period 14% of the weeks had corn silage moving outside this range. Haylage, as expected, was more variable. Close to 50% of the weeks had alfalfa haylage moving more than +/- 3.5 points. No winter forages (wheat, rye, triticale) were included in the study but we speculate that this will vary somewhere in the middle of the corn silage and haylage.
Applied Implications
- Proper sampling technique is required to get a truly representative sample. This is not as difficult as it sounds, but taking one or two handfuls from the face isn’t correct.
- Frequent sampling of ensiled feeds for DM is important. We recommend that silages be tested 2X week. This doesn’t mean that the silage DM in your feeding program needs to be changed each time. Keeping a log of the moisture is important to identify trends. Here is an example string of DM for a few weeks. Corn Silage – 33, 33, 32, 33, 34, 32, 32, 32, 31, 33. In this string we would suggest that ingredient DM not be changed. Haylage – 44, 41, 44, 46, 42, 42, 46, 45, 47, 45. Assume that the DM began at 44. For the first 3 weeks (6 samples) it would be hard to keep up with variation, and we would recommend more frequent drying. Note that the early 46 may have resulted in a move but the next samples were back in line. Later in the period we would suggest that the DM be raised to 45 or 46.
- During pile changes daily testing should be considered. Again, only update the ingredient if the trend is evident.
- The changes observed in this study were observed without major weather events. So during rain, or fog, it is likely beneficial to have an alternate plan in place.
- The final factor to consider is how important accurate weighing of ingredients into the mixer is for diet performance. A string of 400 cows, formulated to receive 15 lbs as fed haylage, will get 6,000 lbs per day. If we are off by 6 points on dry matter this means we will be off by 360 lbs of dry matter to the string. Similarly, if we aren’t off by 6 points, yet we make the ingredient edit, the TMR is still off 360 lbs of dry matter. This example highlights how accurate weighing in combination with accurate DM is required for proper ration preparation.
Study # 2
Ben Abdelkrim, A. et al. 2021. Exploring simultaneous perturbation profiles in milk yield and body weight reveals a diversity of animal responses and new opportunities to identify resilience proxies. J Dairy Sci 104:459-470. (France)
Definitions
Perturbation profiles – Drops in milk yield and/or body weight due to a physical (disease, feeding) or psychological (moves, handling stress) impact, alone or in combination.
Resiliency – The capacity of the animal to be minimally affected by disturbances or to rapidly return to the previous state before exposure.
Quantile – a subset of animals that would be expected to respond in a similar manner.
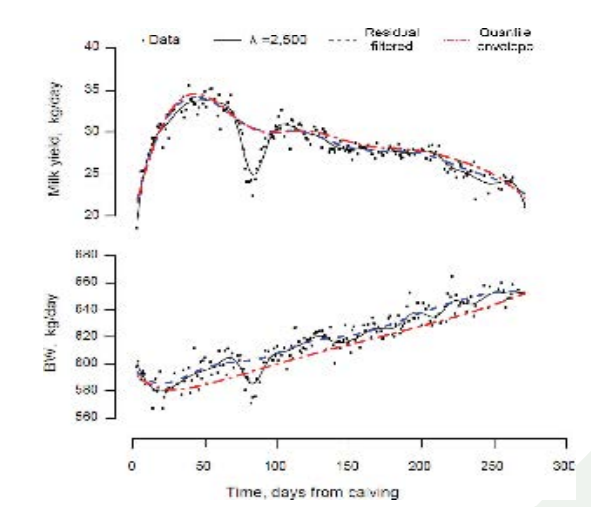
While the study was designed to justify electronic monitoring devices for dairy management, much of the information is applicable to all dairies. This graph (Figure 2 from the paper, shown below) clearly shows the impact of some stress event on performance. At about 70 DIM something happened to “perturb” milk. The black line is the actual milk curve. The blue line shows what was likely to happen without the stress. The red line is showing what happened within a subset of the data of animals that would react similarly. For this newsletter we just want to point out the difference between the blue line “normal” and the black line “stressed” milk yield. What this paper doesn’t identify is causes of the disruption. While this would be helpful, our use of the paper is to show that a stress has immediate, abrupt impact on performance.
Applied Implications
- Stress is never good.
- Identifying stress sooner minimizes the drop in performance
- Animal response, and resiliency, is very individual cow based.
Study # 3
Adriaens, I. et al. 2021. Milk losses and dynamics during perturbations in dairy cows differ with parity and lactation stage. J Dairy Sci. 104:405-418. (Belgium) This study, similar to #2, was a data review from automated milking systems in Belgium. The results confirm what you observe on your dairies. Early lactation cows seem to react quicker and more severely to stress than cows later in lactation. Reasons for the slower drop in milk later are likely because energy demand has already switched from milk production to BW gain, fetal growth, as well as late-lactation immune system challenges, such as different causes of mastitis, have less impact. Similarly, first lactation animals are impacted less than mature cows; likely due to the large demand for energy going to growth and development.
Applied Implications
- Stress is never good.
- Same stress events do not necessarily have the same impact on all animals. There is likely a genetic component to the impact on the animal, and its response. This is evident to anyone that routinely handles cattle.
Summary
We chose these three studies as examples of why consistency is a good management concept. Dry matter determination is the primary on-farm item affecting diet formulation. The two studies on impact of changes just highlight the importance of consistency. In the graph we pulled from the study, the drop in milk at about 70 DIM could easily have been due to a silage change. Most changes are perceived as stress to the animal. Moves during the first 100 DIM need to be well thought out. Extended lock up times have an impact. A “non-formulated” ration change due to loading error will have an impact. All of these factors “perturb” milk yield. While the cows may respond differently to the same stress, how quickly we intervene often determines the magnitude and duration of the drop.